Project 1: Multi-omics Data Integration to Improve Disease Outcome Prediction and Biomarker Identification
Molecular signatures of the genome, transcriptome, and proteome in the cells characterize phenotypes and their alterations are associated with phenotypic changes. Although such molecular signatures can be profiled at the omics level, the phenotypic interpretations and predictions based on these omics profilings are still challenging. Current omics approaches extract simple quantitative information such as gene expression or mutations in a biological model and try to link such one-dimensional information to phenotypes directly. These approaches do not consider multilayered complexity (cis and trans) in the regulatory pathways in cells and tissues. These problems generally produce weak correlations between omics data and phenotypes. For example, gene expression analysis by RNA-seq data does not correlate to the abundance of proteins in the proteome which is critical in determining phenotypes. To overcome these issues, multi-omics approaches have been highlighted. In multi-omics, generally, profiling data from different resources are integrated to identify more powerful molecular signatures that would not be defined in single omics data analysis. Such simple integrative approaches provide a better resolution in data analyses, but they are still weak in defining causative signatures in phenotypes. In this project, a new concept in multi-omics data analyses is developed by considering multi-dimensional parameters in regulatory biology. The regulatory parameters includes cis-elements in RNA and their regulatory interactions with trans-acting molecules. Thus, our goals are to systemically develop multi-omics models that simulate the regulatory interaction networks at the transcriptome level and predict the proteome phenotype.
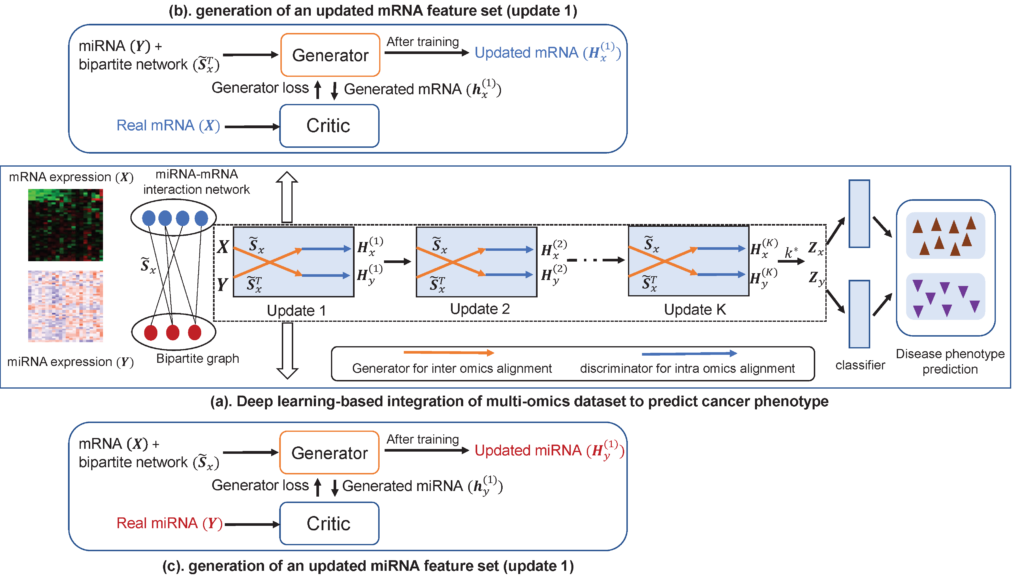
- Sudipto Baul, Khandakar Tanvir Ahmed, Qibing Jiang, Guangyu Wang, Qian Li, Jeongsik Yong, and Wei Zhang#. Integrating Spatial Transcriptomics and Bulk RNA-seq: Predicting Gene Expression with Enhanced Resolution through Graph Attention Networks. Briefings in Bioinformatics, 2024. (#Corresponding author) doi:10.1093/bib/bbae316 [Download]
- Khandakar Tanvir Ahmed, Sudipto Baul, Yanjie Fu, and Wei Zhang#. Attention-Based Multi-modal Missing Value Imputation for Time Series Data with High Missing Rate. SIAM International Conference on Data Mining (SDM), April 2023. (#Corresponding author) 10.1137/1.9781611977653.ch53
- Khandakar Tanvir Ahmed, Sze Cheng, Qian Li, Jeongsik Yong, and Wei Zhang#. Incomplete Time-Series Gene Expression in Integrative Study for Islet Autoimmunity Prediction. Briefings in Bioinformatics, 2022. (#Corresponding author) doi:10.1093/bib/bbac537 [Download]
- Khandakar Tanvir Ahmed, Jiao Sun, Sze Cheng, Jeongsik Yong, and Wei Zhang#. Multi-omics Data Integration by Generative Adversarial Network. Bioinformatics, 2021. (#Corresponding author) doi:10.1093/bioinformatics/btab608 [Download]
- Jiao Sun, Jae-Woong Chang, Teng Zhang, Jeongsik Yong, Rui Kuang, and Wei Zhang#. Platform-integrated mRNA Isoform Quantification. Bioinformatics, 2020. (#Corresponding author) doi:10.1093/bioinformatics/btz932 [Download]
- Wei Zhang, Raphael Petegrosso, Jae-Woong Chang, Jiao Sun, Jeongsik Yong, Jeremy Chien, and Rui Kuang. A Large-Scale Comparative Study of Isoform Expressions Measured on Four Platforms. BMC Genomics, 2020. doi:10.1186/s12864-020-6643-8 [Download]
____________________________________________________________________________________________________________
Project 2: Computational Methods to Explore the Role of Post-transcriptional Regulation in Cancer
Deregulation of gene expression is a hallmark of human tumor cells. Post-transcriptional regulation is a pervasive mechanism in the regulation of most human genes, its implication in cancer is only beginning to be appreciated. Exploring the complex causal relations in post-transcriptional regulation can be one of the most effective and efficient ways to improve the understanding of cancer and assess the cause of disease for new patients with already collected data influencing. This project investigates the role of post-transcriptional regulation in cancer patients with mRNA sequencing (RNA-seq) data, microRNA sequencing (miRNA-seq) data, and microRNA-mRNA interaction network in three folds: 1) developing advanced machine learning methods to identify alternative polyadenylation (APA) events in the 3’ untranslated region (3’UTR) of the mRNAs; 2) developing biologically motivated graph-based learning models and efficient scalable algorithms to estimate the protein expression levels; 3) investigating the prognostic power of the identified APA and estimated protein expressions.
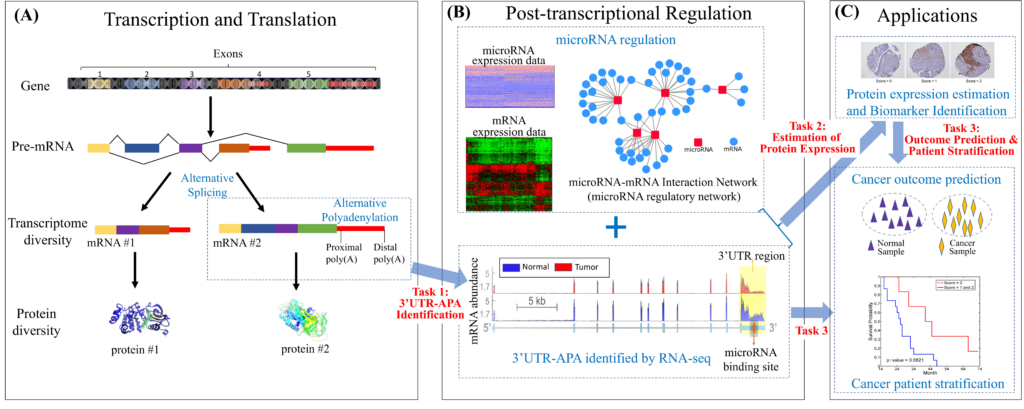
- Naima Ahmed Fahmi, Khandakar Tanvir Ahmed, Jae-Woong Chang, Heba Nassereddeen, Deliang Fan, Jeongsik Yong, and Wei Zhang#. APA-Scan: Detection and Visualization of 3′-UTR APA with RNA-seq and 3′-end-seq Data. BMC Bioinformatics, 2022. (#Corresponding author) doi:10.1186/s12859-022-04939-w [Download]
- Sze Cheng*, Naima Ahmed Fahmi*, Meeyeon Park, Jiao Sun, Kaitlyn Thao, Hsin-Sung Yeh, Wei Zhang#, and Jeongsik Yong#. mTOR contributes to the proteome diversity through transcriptome-wide alternative splicing. International Journal of Molecular Sciences, 2022.
- Khandakar Tanvir Ahmed*, Jiao Sun*, William Chen, Irene Martinez, Sze Cheng, Wencai Zhang, Jeongsik Yong, and Wei Zhang#. In Silico Model for miRNA-mediated Regulatory Network in Cancer. Briefings in Bioinformatics, 2021. (#Corresponding author) doi.org/10.1093/bib/bbab264 [Download]
- Naima Ahmed Fahmi, Heba Nassereddeen, Jae-Woong Chang, Meeyeon Park, Hsin-Sung Yeh, Jiao Sun, Deliang Fan, Jeongsik Yong#, and Wei Zhang#. AS-Quant: Detection and Visualization of Alternative Splicing Events with RNA-seq Data. International Journal of Molecular Sciences, 2021. (#Corresponding author) doi.org/10.3390/ijms22094468 [Download]
- Jiao Sun*, Naima Ahmed Fahmi*, Heba Nassereddeen, Sze Cheng, Irene Martinez, Deliang Fan, Jeongsik Yong, and Wei Zhang#. Computational Methods to Study Human Transcript Variants in COVID-19 Infected Lung Cancer Cells. International Journal of Molecular Sciences, 2021. (#Corresponding author) doi:10.3390/ijms22189684
- Jae-Woong Chang, Hsin-Sung Yeh, Meeyeon Park, Luke Erber, Jiao Sun, Sze Cheng, Alexander M. Bui, Naima Ahmed Fahmi, Ryan Nasti, Rui Kuang, Yue Chen, Wei Zhang#, and Jeongsik Yong#. mTOR-regulated U2af1 tandem exon splicing specifies transcriptome features for translational control. Nucleic Acids Research, 2019. (#co-corresponding authors) doi:10.1093/nar/gkz761
- Jae-Woong Chang*, Wei Zhang*, Hsin-Sung Yeh, Meeyeon Park, Chengguo Yao, Yongsheng Shi, Rui Kuang#, and Jeongsik Yong#. An Integrative Model for Alternative Polyadenylation, IntMAP, Delineates mTOR-modulated Endoplasmic Reticulum Stress Response. Nucleic Acids Research, 2018. (*Joint first authors) doi:10.1093/nar/gky340 [Download]
_____________________________________________________________________________________________________
Project 3: Cancer Drug Sensitivity Estimation with Brightfield Time-lapse Microscopy Imaging
Time-lapse microscopy is a powerful technique that relies on images of live cells cultured ex vivo that are captured at regular intervals of time to describe and quantify their behavior under certain experimental conditions. This imaging method has great potential in advancing the field of precision oncology by quantifying the response of cancer cells to various therapies and identifying the most efficacious treatment for a given patient. Digital image processing algorithms developed so far require high-resolution images involving very few cells originating from homogeneous cell line populations. We propose a novel framework that tracks cancer cells to capture their behavior and quantify cell viability to inform clinical decisions in a high-throughput manner. The brightfield microscopy images a large number of patient-derived cells in an ex vivo reconstruction of the tumor microenvironment treated with 31 drugs for up to 6 days. We developed robust and user-friendly pipelines that detect cells in co-culture, track these cells across time, and identify cell death events using changes in cell attributes.
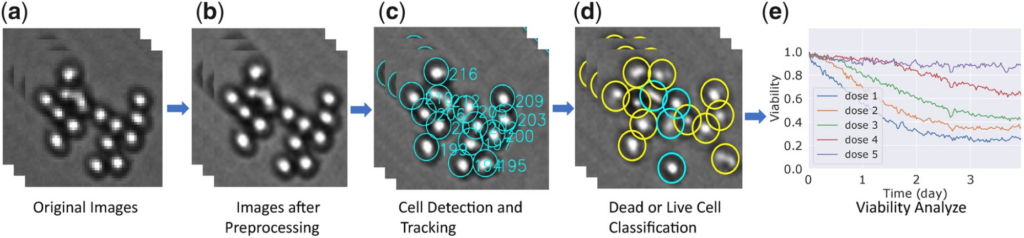
- Qibing Jiang, Praneeth Reddy Sudalagunta, Mark Meads, Xiaohong Zhao, Alexandra Achille, David Noyes, Maria Silva, Rafael Renatino Canevarolo, Ken Shain, Ariosto Silva, and Wei Zhang#. TLCellClassifier: Machine Learning Based Cell Classification for Bright-Field Time-Lapse Images. BioRxiv
- Qibing Jiang, Praneeth Sudalagunta, Maria C. Silva, Rafael R. Canevarolo, Xiaohong Zhao, Khandakar Tanvir Ahmed, Raghunandan Reddy Alugubelli, Gabriel DeAvila, Alexandre Tungesvik, Lia Perez, Robert Gatenby, Robert Gillies, Rachid Baz, Mark B. Meads, Kenneth H. Shain, Ariosto S. Silva, and Wei Zhang#. CancerCellTracker: A Brightfield Time-lapse Microscopy Framework for Cancer Drug Sensitivity Estimation. Bioinformatics, 2022. doi:10.1093/bioinformatics/btac417 [Download]
- Qibing Jiang, Praneeth Sudalagunta, Mark B. Meads, Khandakar Tanvir Ahmed, Tara Rutkowski, Ken Shain, Ariosto S. Silva, and Wei Zhang#. An Advanced Framework for Time-lapse Microscopy Image Analysis. BioRxiv
_____________________________________________________________________________________________________
Project 4: Network-based Machine Learning and Graph Theory Algorithms for Precision Oncology
Our lab has developed a set of graph-based learning models for building predictive models and mining biomarkers of disease phenotypes from high-throughput sequencing data and protein-protein interaction networks. Each model formulates one unified learning framework to retrieve the global structures of the networks and capture the molecular organization in the cellular system. The comprehensive evaluation of these models shows that they lead to considerable improvements over standard learning models and reveal subnetwork signatures for predicting outcomes of disease treatments.
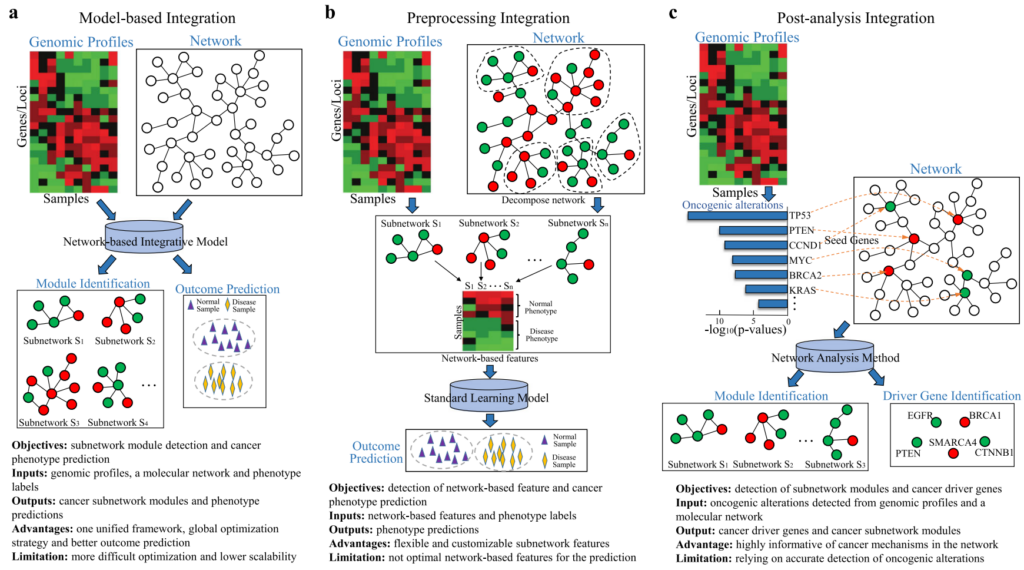
- Sudipto Baul, Khandakar Tanvir Ahmed, Joseph Filipek, and Wei Zhang#. omicsGAT: Graph Attention Network for Cancer Subtype Analyses. International Journal of Molecular Sciences, 2022. doi:10.3390/ijms231810220 [Download]
- Khandakar Tanvir Ahmed, Sunho Park, Qibing Jiang, Taehyun Hwang, and Wei Zhang#. Network-based Drug Sensitivity Prediction. International Conference on Intelligent Biology and Medicine (ICIBM), August 2020. BMC Medical Genomics, 2020. (#Corresponding author) doi:10.1186/s12920-020-00829-3 [Download]
- Zhibo Wang, Zhezhi He, Milan Shah, Teng Zhang, Deliang Fan, and Wei Zhang#. Network-based Multi-Task Learning Models for Biomarker Selection and Cancer Outcome Prediction. Bioinformatics, 2020. (#Corresponding author) doi:10.1093/bioinformatics/btz809 [Download]
- Wei Zhang, Jae-Woong Chang, Lilong Lin, Kay Minn, Baolin Wu, Jeremy Chien, Jeongsik Yong, Hui Zheng, and Rui Kuang. Network-based Isoform Quantification with RNA-Seq Data for Cancer Transcriptome Analysis. PLoS Comput Biol, 2015. doi:10.1371/journal.pcbi.1004465 [Download]
- Wei Zhang, Takayo Ota, Viji Shridhar, Jeremy R Chien, Baolin Wu, and Rui Kuang. Network-based Survival Analysis Reveals Subnetwork Signatures for Predicting Outcomes of Ovarian Cancer Treatment. PLoS Comput Biol, 2013. doi:10.1371/journal.pcbi.1002975 [Download]
- Wei Zhang, Nicholas Johnson, Baolin Wu, and Rui Kuang. Signed Network Propagation for Detecting Differential Gene Expressions and DNA Copy Number Variations. Proc. of ACM Conference on Bioinformatics, Computational Biology and Biomedicine (ACM BCB), Oct 2012. [Paper][Download]
_____________________________________________________________________________________________________
Project 5: Fast and Efficient DNA Sequence Alignment in Non-Volatile Magnetic RAM
The state-of-the-art DNA sequencing technologies could generate Terabytes of DNA sequence data in a single run, and their throughput is expected to increase 3-5 times each year in the coming years. In order to apply these big DNA data to follow-up complex disease diagnostics/prognostics, such as cancer risk assessment, tailor patient treatment, and prenatal testing, they must be first aligned to a 3.2-billion-length human reference genome. However, the existing software tools for this purpose may need hours or days to align such large amounts of DNA sequence data even with very powerful computing systems of today due to the ‘memory wall’ challenge in state-of-the-art computing architecture that describes the speed mismatch between memory units and computing units. To end this, the project leverages innovations from non-volatile nano-magnet-based Magnetic Random Access Memory (MRAM) technology and in-memory computing architecture. It achieves up to two orders of magnitude higher computing performance, speed, and energy efficiency for next-generation DNA sequence analysis systems, which enables large-scale fast genomic data analytics to support research on various disease studies and biomedical applications. This project follows two main research tracks. The first one explores how to leverage the intrinsic non-volatile MRAM device property to efficiently develop ultra-parallel, reconfigurable in-memory logic required by DNA alignment computation and its big DNA-data Processing-in-Memory (PIM) accelerator architecture. The second research track investigates how to develop fast DNA alignment-in-memory algorithm based on Burrows-Wheeler Transformation to match with the proposed MRAM-based PIM platform and its large-scale genomic analysis application in disease phenotype prediction. Alignments generated are applied to estimate gene expression, and identify single nucleotide mutation events for patient samples, leading to molecular signatures for disease risk assessment.
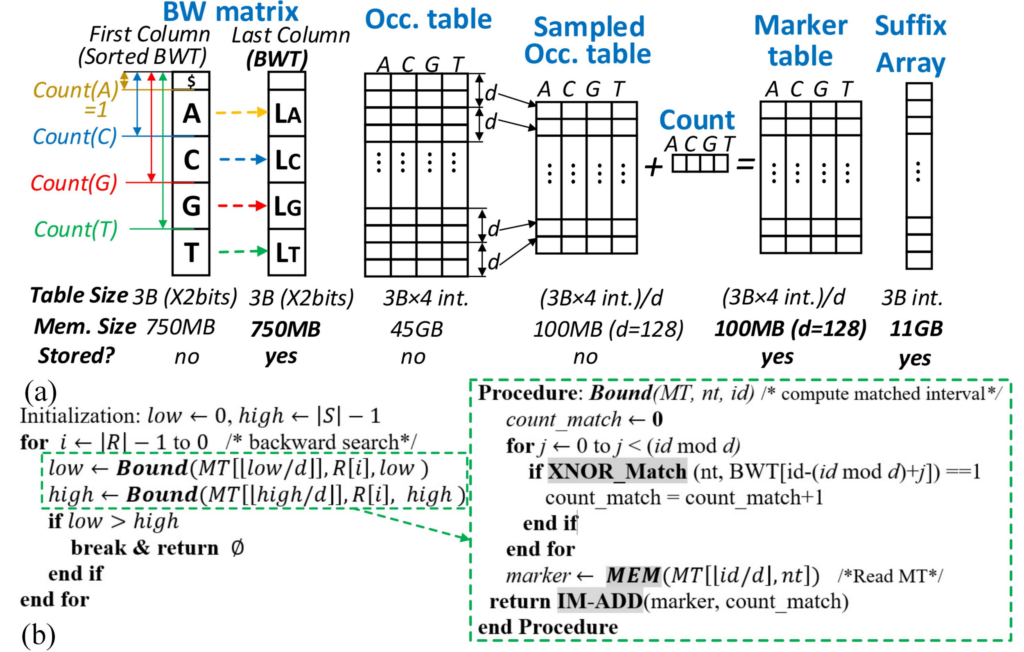
- Fan Zhang, Amitesh Sridharan, Wangxin He, Injune Yeo, Maximilian Liehr, Wei Zhang, Nathaniel Cady, Yu Cao, Jae-Sun Seo, and Deliang Fan. A 65-nm RRAM Compute-in-Memory Macro for Genome Processing. IEEE Journal of Solid-State Circuits, 2024. 10.1109/JSSC.2024.3396429
- Shaahin Angizi, Naima Ahmed Fahmin, Deniz Najafi, Wei Zhang, and Deliang Fan. PANDA: Processing in Magnetic Random-Access Memory-Accelerated de Bruijn Graph-Based DNA Assembly. Journal of Low Power Electronics and Applications, 2024. 10.3390/jlpea14010009
- Fan Zhang, Shaahin Angizi, Jiao Sun, Wei Zhang, and Deliang Fan. Aligner-D: Leveraging in-DRAM Computing to Accelerate DNA Short Read Alignment. IEEE Journal on Emerging and Selected Topics in Circuits and Systems, 2023. 10.1109/JETCAS.2023.3241545
- Fan Zhang, Shaahin Angizi, Naima Ahmed Fahmi, Wei Zhang, and Deliang Fan. PIM-Quantifier: A Processing-in-Memory Platform for Genome Quantification. Design Automation Conference (DAC), Dec 2021. doi: 10.1109/DAC18074.2021.9586144
- Shaahin Angizi, Wei Zhang, and Deliang Fan. Exploring DNA Alignment-in-Memory Leveraging Emerging SOT-MRAM. Great Lakes Symposium on VLSI, Sept 2020. doi:10.1145/3386263.3407590
- Shaahin Angizi, Naima Ahmed Fahmi, Wei Zhang, and Deliang Fan. PIM-Assembler: A Processing-in-Memory Platform for Genome Assembly. Design Automation Conference (DAC), July 2020. doi:10.1109/DAC18072.2020.9218653
- Shaahin Angizi, Jiao Sun, Wei Zhang, and Deliang Fan. PIM-Aligner: A Processing-in-MRAM Platform for Biological Sequence Alignment. Design, Automation and Test in Europe (DATE), Mar 2020. doi:10.23919/DATE48585.2020.9116303
- Shaahin Angizi, Jiao Sun, Wei Zhang, and Deliang Fan. AlignS: A Processing-In-Memory Accelerator for DNA Short Read Alignment Leveraging SOT-MRAM. Design Automation Conference (DAC), June 2019. doi:10.1145/3316781.3317764
- Shaahin Angizi, Jiao Sun, Wei Zhang, and Deliang Fan. GraphS: A Graph Processing Accelerator Leveraging SOT-MRAM. Design, Automation and Test in Europe (DATE), Mar 2019. doi:10.23919/DATE.2019.8715270
_____________________________________________________________________________________________________
Funding
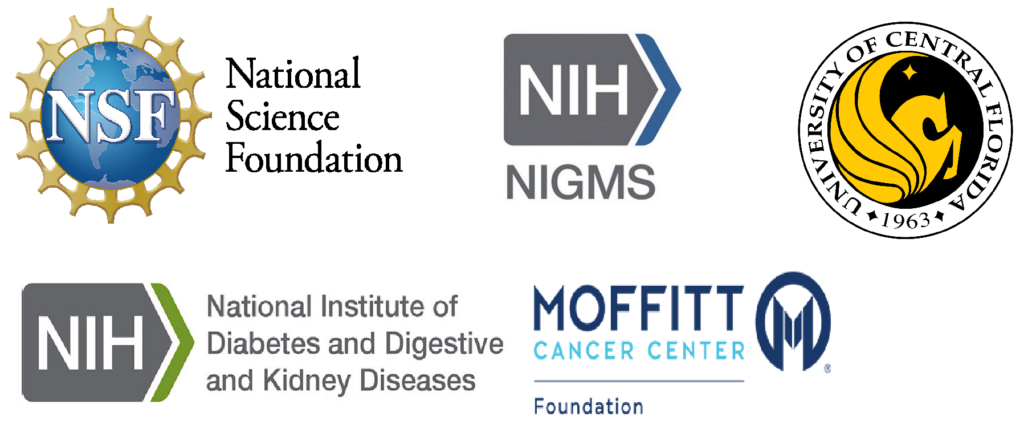
omicsGAT
Graph Attention Network for Cancer Subtype Analyses
CancerCellTracker
A Brightfield Time-lapse Microscopy Framework for Cancer Drug Sensitivity Estimation
APA-Scan
Detection and Visualization of 3′-UTR APA with RNA-seq and 3′-end-seq Data
omicsGAN
Multi-omics Data Integration by Generative Adversarial Network
PTNet
In silico model for miRNA-mediated regulatory network in cancer
Incomplete Time-Series Gene Expression in Integrative Study
AS-Quant
Detection and Visualization of Alternative Splicing Events with RNA-seq Data
Graph-based Drug Sensitivity Prediction
PTNet
Platform-integrated mRNA Isoform Quantification
NetML
Network-based Multi-Task Learning Models for Biomarker Selection and Cancer Outcome Prediction
IntMAP
An Integrative Model for Alternative Polyadenylation